

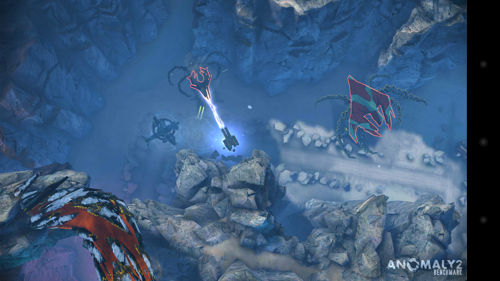
Emmott, Andrew Das, Shubhomoy Dietterich, Thomas Fern, Alan Wong, Weng-Keen (2016): Anomaly Detection Meta-Analysis Benchmarks.The intended contributions of this article are many in addition to providing a large publicly-available corpus of anomaly detection benchmarks, we provide an ontology for describing anomaly detection contexts, a methodology for controlling various aspects of benchmark creation, guidelines for future experimental design and a discussion of the many potential pitfalls of trying to measure success in this field. Last, we compare results against a trivial solution as an alternate means of normalizing the reported performance of algorithms. We then offer several approaches to summarizing our experimental results, drawing several conclusions about the impact of our methodology as well as the strengths and weaknesses of some algorithms. We employ statistical hypothesis testing to demonstrate the value (or lack thereof) of our benchmarks. Second, results are evaluated with two metrics, ROC Area Under the Curve and Average Precision.
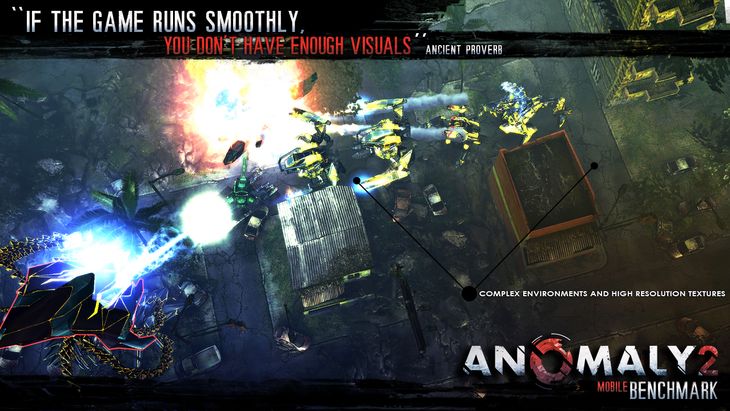
First we observe the effects of experimental design on experimental results. We analyze these results to understand many phenomena observed in previous work. We apply a representative set of anomaly detection algorithms to this corpus, yielding a very large collection of experimental results. To accomplish this we first identify approaches to benchmarking anomaly detection algorithms across the literature and produce a large corpus of anomaly detection benchmarks that vary in their construction across several dimensions we deem important to real-world applications: (a) point difficulty, (b) relative frequency of anomalies, (c) clusteredness of anomalies, and (d) relevance of features. This article provides a thorough meta-analysis of the anomaly detection problem.Citeable URL: Descriptions Attribute Name
